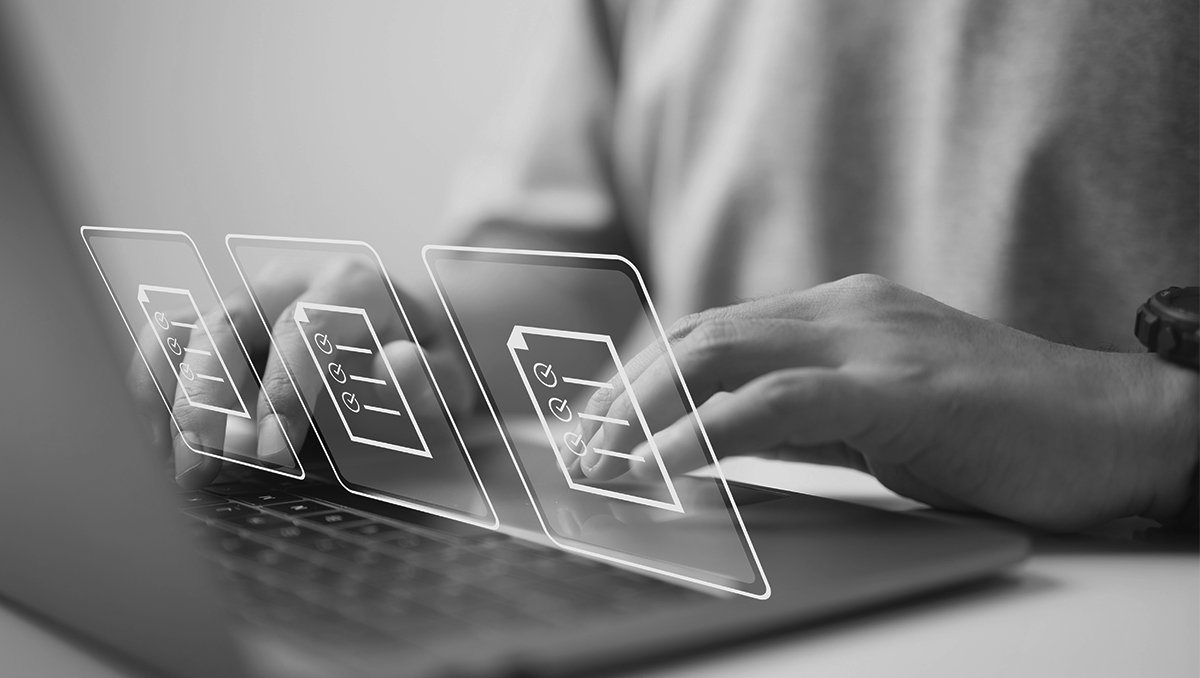
Organizations must constantly make decisions about their data. This can be challenging, especially given today’s high-frequency volume and variety.
Data classification guidelines provide a structure for managing, protecting, and utilizing data effectively in an organization. By following the guidelines enumerated in this article, organizations can ensure a functional and effective data classification system to protect their sensitive data and maintain regulatory compliance.
Data Classification Guidelines:
Data classification guidelines assist organizations in managing and protecting their data cost-effectively and efficiently, in accordance with their business requirements and the levels of sensitivity and confidentiality. These guidelines involve:
- Identifying Data: This involves understanding the types of data held and processed within the organization, such as customer, employee, business operations, intellectual property, and financial data.
- Categorizing Data: After identifying the data, they must be categorized based on their sensitivity. The common categories are public, internal, confidential, and highly confidential.
- Labeling or Tagging Data: This process involves tagging each data set according to its category to enable easier identification and appropriate control.
- Implementing Controls: Relevant security controls should be implemented based on the categorization. For example, Public data may require no specific protection, whereas highly confidential data may need strong encryption and strict access controls.
- Training Employees: All employees should be trained on the data classification scheme and the controls necessary for each category.
- Reviewing the Process: The data classification process should be reviewed and updated periodically to address changes in business requirements, regulatory landscape or the data itself.
Data Classification Techniques:
- Content-based Classification: This method involves classifying data based on the content within files, which can be highly accurate but resource-intensive.
- Context-based Classification: This method considers the metadata (e.g., location, creator, date of creation) related to the data to determine its classification.
- User-based Classification: In this method, users are entrusted with classifying the data they generate or manage. While this can be subjective and inconsistent, it can also be more accurate, as the users are typically the most aware of the data’s sensitivity.
- Machine Learning Classification: Advanced systems use machine learning algorithms to classify data based on patterns and trends, improving speed and accuracy. However, it may require significant technical expertise to set up and manage.
- Hybrid Classification: This approach combines different classification techniques to enhance the accuracy and efficiency of the overall process.
How Does Data Classification Guidelines Work?
Data classification guidelines outline the steps and processes an organization must follow to categorize and manage its data effectively.
The guidelines usually include processes such as:
- Creating Classification Levels: The first step in data classification guidelines usually involves creating various classification levels based on the data's sensitivity. Common classification levels include public, internal, confidential, and restricted.
- Identifying Data Types: This involves identifying the types of data the organization handles. This could include personally identifiable information, financial data, intellectual property, etc.
- Assigning Classification Levels: Once the data types have been identified, they are then assigned to the appropriate classification levels based on their sensitivity and importance to the organization.
- Developing Security Measures: Specific security measures are implemented based on the data classification level. More sensitive data typically requires stronger security measures, such as encryption or limited access permissions.
- Training Staff: Guidelines usually include training staff on the data classification so they understand how to handle the data accordingly.
- Implementing Data Lifecycle Management: This involves establishing procedures for data retention, access, use, and disposal based on its classification, which can involve archiving or permanently deleting data once it is no longer needed.
- Conducting Regular Reviews: The guidelines typically call for regular reviews and updates of the data classification process to ensure it stays relevant as the organization evolves and possibly deals with new types of data.
What Are the Advantages of Data Classification Guidelines?
- Enhanced Data Security: Data classification guidelines help organizations identify their most crucial and sensitive data, ensuring robust security controls are in place to prevent data breaches or leaks.
- Regulatory Compliance: Adherence to data classification guidelines helps organizations meet regulatory requirements such as those outlined by the GDPR, CCPA, HIPAA, and more, avoiding hefty penalties.
- Data Governance: Effective data governance requires a good understanding of the types of data an organization collects and maintains. Data classification techniques offer insights needed to create appropriate data usage policies.
- Efficient Use of Resources: With clear classification guidelines, organizations can focus their resources on securing high-priority data, ensuring efficiency.
- Improved Data Management: Guidelines make it easier for organizations to manage and organize vast amounts of data, making data retrieval, backup, or transfers more efficient.
- Facilitated Decision-Making: When data is classified, decision-making becomes easier and faster because the value of information is known.
- Risk Management: Identifying the sensitivity of data helps organizations understand their risk exposure and work towards mitigating these risks.
- Data Life-cycle Management: Classification guidelines make it easier to determine how long to retain specific data types, or when it's time to destroy or archive them in accordance with industry and legal data retention requirements.
- Better Employee Understanding: Guidelines clearly explain data sensitivity labels and their associated levels, helping employees handle data appropriately and responsibly.
- Facilitates Incident Response: In case of a security incident, already having classified data allows for quicker, more efficient responses.
- Vendor Management: Information about data sensitivity can be communicated to third-party vendors handling your data, ensuring they adhere to required protocols.
- Informed IT Planning and Investments: Understanding data classifications can inform decisions about IT infrastructure planning and investments, like data storage and security solutions.
What Are the Disadvantages of Data Classification Guidelines?
- Complexity: The data classification process can be quite complex, especially for large organizations with vast data volumes.
- High Implementation Cost: Implementing data classification processes might require considerable resources and investment in specialized tools and technical expertise.
- Time Consumption: Effective data classification can be time-consuming, especially if done manually.
- Misclassification Risk: There's a risk that data could be misclassified either due to human error or limitations in classification tools. This could result in insufficient protections for sensitive data or unnecessary security measures applied to less important data.
- Maintenance Challenge: Data classification isn't a one-time process. It needs to be routinely reviewed and updated based on changes in data usage, regulatory laws, technology, organization policies, etc., which can be challenging.
- User Resistance: Employees might resist the data classification process because classification restricts data handling and sharing.
- Dependency on Expertise: The efficiency of data classification often depends on the expertise and understanding of the person doing the classification.
- Privacy Issues: Data classification could potentially infringe on data privacy, especially if personal data are being classified and handled by multiple entities in the organization.
- Overclassification: Sometimes, organizations may overclassify data, applying strict controls even when unnecessary, causing unnecessary burdens and inefficiencies.
What Are the Best Practices of Data Classification Guidelines?
Best practices for creating data classification guidelines include:
- Formulate a clear policy: A well-defined data classification policy is the foundation of data classification. The policy should include goals, steps to classify data, the scope, responsibilities of individuals, and consequences of non-compliance.
- Identify data types: It's crucial to identify what types of data your organization deals with. This could include customer information, financial data, health records, and others. Knowing what data you have is the first step towards effectively classifying it.
- Establish clear classification categories: Classification categories should clearly reflect the type and sensitivity of data. Categories may include public, internal, confidential, and top-secret.
- Use automated tools: Automation can help classify large volumes of data accurately and efficiently, eliminating human error. It can also help maintain classification schemas as data grows or changes over time.
- Conduct regular reviews and audits: Ensure your classification policy is constantly updated and fine-tuned. Regular audits can help identify mislabeled data, gaps in policy, or new data that requires classification.
- Return on investment: Remember, the goal of data classification is to enhance security, reduce risk, and operational efficiency. Hence, ensure the process contributes positively to your organization's return on investment.
- Training and awareness: Ensure all employees understand data classification's importance. Regular training can reinforce the various classification categories, what data falls into each, and the consequences of misclassification.
- Collaborate and communicate: Successful data classification requires collaboration across different departments. Every member should understand and abide by the data classification policy from IT to legal to HR.
- Document Everything: Documentation serves as a manual for employees, promotes and enables compliance, and provides evidence of your efforts to secure and protect data if auditors or regulators ever request it.
- Encryption: Encrypt sensitive data whenever possible to offer an additional layer of security. This is especially important for confidential data that could cause significant harm if accessed by unauthorized entities.
- Integration with other security measures: Data classification should not work in isolation. It should be integrated with other data protection measures, such as Data Loss Prevention (DLP) tools, identity and access management (IAM), and intrusion detection systems (IDS).
Why You Should Embrace Fortra’s Data Classification
Organizations can strengthen their data protection and security, streamline operations, and ensure compliance with regulations.
Fortra understands that the choice of data classification method depends on the organization's specific needs and resources, and we have the insight to guide you accordingly.
Request a demo to see our data classification in action.