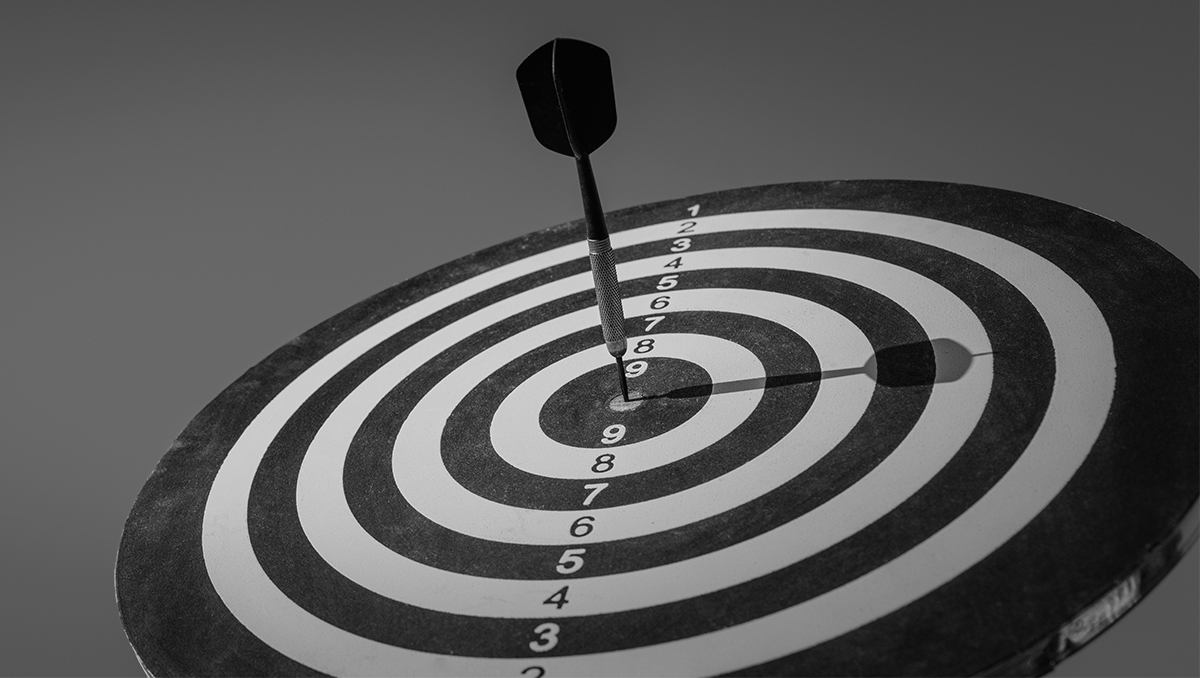
Not every organization can afford to engage in a data arms race to remain competitive in the data-hungry era of generative AI and large language models. However, embracing data classification best practices is a cost-effective way to get the desired outcomes from your data, highlighting its important value, risk sensitivity, and compliance requirements. Data classification best practices involve a systematic approach to categorizing data based on its sensitivity, importance, and regulatory requirements
What Are Data Classification Best Practices?
Data classification best practices involve a systematic approach to categorizing data based on its sensitivity, importance, and regulatory requirements
Here are some key best practices:
- Define Clear Objectives: Understand why you’re classifying data and what results you expect.
- Create a Data Classification Policy: Establish a policy defining what data falls into which category, determine who is responsible for classifying new data, and outline how to handle data as it moves from one category to another.
- Understand Regulatory Requirements: Your data classification strategy should account for any and all compliance requirements related to data handling, storage, and distribution.
- Use Standardized Classification Levels: Standard classification levels, such as public, internal, confidential, and restricted, can help maintain consistency.
- Consistency across Platforms: Ensure that classification policy and procedures apply consistently across all platforms, devices, and locations.
- Manual vs. Automated Classification: Determine what data sets might require manual classification (e.g., highly sensitive) and where automated tools could be beneficial.
- Staff Training: Employees should be educated on classification definitions, the importance of timely and accurate classification, and the repercussions of misclassifying data.
- Continual Review Process: Data classification is an ongoing process. Regular check-ins are necessary to ensure policy relevance and regulation adherence and to address shifts in business requirements and goals.
- Integrating with Other Security Measures: Classification should not work in silo but integrate with other data security measures like access controls, encryption, and data loss prevention.
- Use of Data Classification Tools: A range of tools are available to support data classification. These include data discovery, data classification, and DLP tools.
The Benefits of Effective Data Classification Best Practices
Implementing effective data classification best practices comes with several benefits:
- Improved data security: By identifying the most sensitive and critical data, organizations can focus on stronger security measures to prevent unauthorized access, breaches, or data loss.
- Regulatory compliance: Many government and industry regulations, such as GDPR, HIPAA, and PCI DSS, require businesses to protect certain types of data. Proper classification ensures data compliance, thus avoiding hefty fines and legal issues.
- More efficient data management: Categorizing data makes it easier to locate specific files, accelerating decision-making and streamlining workflows.
- Better decision-making: Data classification can improve business and data intelligence and help with strategic planning by giving organizations a clearer understanding of their data.
- Reduced data storage costs: By classifying data, organizations can identify obsolete or redundant data, reducing unnecessary storage usage and costs.
- Risk minimization: Organizations can minimize the risks associated with data breaches or losses by identifying sensitive data and applying appropriate protective measures.
- Productivity and efficiency: Automated data classification tools streamline the process of protecting and managing data, freeing up employees' time to focus on more value-adding activities.
- Greater visibility and control: Classification provides insight into the type and location of data within the organization, allowing for better control over internal and external data transfers.
- Facilitates auditability: Classified data simplifies the audit process as auditors can easily identify and access the necessary information.
- Increased end-user awareness: Effective data classification practices can help educate employees about the sensitivity of information, promoting a more security-conscious culture.
The Challenges of Implementing Data Classification Best Practices
Implementing data classification best practices involves several challenges:
- Large Data Volumes: Today, organizations handle massive amounts of data, making data classification daunting. The sheer volume presents logistical and scalability challenges, especially regarding unstructured data.
- Identifying Sensitivity Levels: Determining the sensitivity level of different data types can be complex. Each piece of data must be reviewed carefully to ensure it is categorized accurately.
- Compliance with Multiple Regulations: Many organizations fall under multiple regulatory frameworks like GDPR, HIPAA, PCI-DSS, and more, each with its own standards for data classification. Meeting the requirements of each can be difficult and time-consuming.
- False Positives and Negatives: Automated data classification systems can sometimes produce false positives and negatives, complicating the process and leading to potential security risks.
- Lack of Awareness and Training: Employees often lack awareness about the importance of data classification, compliance regulations, and best practices. This can lead to improper data handling and mishaps.
- Changes in Data: Data can change in value or sensitivity over time or even in different contexts. Keeping up with these changes can be challenging.
- Cost: Implementing data classification practices and systems could require significant resources and investment.
- Keeping Up-to-Date: Regulations, threats, technology, and business needs constantly evolve. Keeping your classification process up to date with these changes can be challenging.
- Legacy Systems: Older systems may not support advanced data classification systems, making it difficult to classify older data or implement a uniform policy across all systems.
The Steps to Data Classification Best Practices
By following these steps, organizations can ensure that they adhere to the best data classification practices.
- Risk Assessment: The first step in implementing data classification best practices is to conduct a comprehensive data risk assessment.
This entails recognizing the data types within your possession, identifying their locations, determining authorized access, and evaluating the protective measures. This should also include an evaluation of potential vulnerabilities and threats. - Define Classification Levels: Identify and define the different levels of data classification, usually as public, internal, confidential, and restricted. Each level should come with a specific set of protective measures.
- Create a Data Inventory: An organization needs to know what data it has in order to classify it correctly. This process includes identifying data types, their locations, who has access to it, and who owns them.
- Develop a Data Classification Policy: Develop a policy that outlines how to classify data, who is responsible for classifying data, and how often data classifications should be reviewed or updated. The policy should also detail the consequences of non-compliance with classification processes.
- Train Employees: All employees should receive general awareness training on data classification. Those directly involved in data handling and management should have more specialized training on classification procedures and requirements.
- Use Classification Tools: Where possible, use automated data classification tools because these can greatly improve the speed and accuracy of data classification.
- Apply Protective Measures: Based on the classification levels, apply the necessary protective measures, such as encryption for highly sensitive data.
- Regularly Audit and Update the Classification Process: Regular audits should be conducted to ensure compliance with the classification policy. The process should also be continually monitored and updated to account for new data types, new regulatory requirements, or changes in the business environment.
- Incident Response Plan: A well-documented response plan should be in place in case of data breaches. This plan aids in swift action to limit damages.
- Review Legal Requirements: Ensure the data classification process meets any legal requirements, particularly those related to sensitive personal data. Compliance with data privacy laws like GDPR and CCPA should be part of the classification protocol.
The Trends and Technologies That Will Impact Data Classification Best Practices in the Future
The following trends and technologies will likely reshape data classification best practices in the future:
- Artificial Intelligence (AI) and Machine Learning (ML): AI and ML algorithms can automate data classification and increase efficiency and accuracy. These technologies can intelligently decipher patterns, analyze behaviors, predict user needs, and handle extensive volumes of data much faster than humans.
- Internet of Things (IoT): The rapid expansion of IoT will result in an unprecedented volume of data generated from various devices. Future data classification practices must accommodate this massive influx of diverse data.
- Blockchain: Blockchain’s tamper-proof ledger system can significantly upgrade data classification privacy, security, and traceability, making each transaction transparent and secure. This could influence how data is classified, especially in industries that require high data integrity, such as finance or healthcare.
- Cloud Computing: As more businesses move to the cloud, efficient data classification will become crucial to managing data effectively in cloud-based storage systems. It must adapt to the dynamic nature of data in the cloud while maintaining data security and privacy.
- Big Data: The growth of Big Data will push for advanced data classification tools to handle the vast amounts of data and extract valuable insights in real-time. Handling this data will require automated, updated, and regulated classification algorithms.
- Data Privacy Regulations: The continual evolution of data protection laws, such as the General Data Protection Regulation (GDPR), California Consumer Privacy Act (CCPA), etc., will continue to influence data classification practices directly. Businesses must maintain data that complies with the latest regulations, which will involve regularly updating and auditing their data classification practices.
- Quantum Computing: Although still in its early stages, quantum computing has the potential to revolutionize data classification by processing large amounts of data at an unprecedented speed. Once it becomes more mainstream, it could vastly affect how businesses classify their data.
- Data Fabric: This is a new approach to data integration that creates a unified, integrated view of data across the enterprise, allowing agile access, delivery, and processing. The increased adaptation of the data fabric will certainly impact the future of data classification.
Learn How Fortra's Data Classification Best Practices Can Help
While data classification appears straightforward in theory, it is difficult to implement accurately. You need a partner like Fortra, who understands the importance of using a content’s context to drive repeatability and predictability in your data classification.
Schedule a demo with us to observe how our data classification works.